Computer vision is an emerging technology in which Artificial Intelligence (AI) reads and interprets images or videos, and then provides that data to decision makers. For the transportation field, computer vision has broad implications, streamlining many tasks that are currently performed by staff. By automating monitoring procedures, transportation agencies can gain access to improved, real-time incident data, as well as new metrics on traffic and “near-misses,” which contribute to making more informed safety decisions.
To learn more about the how computer vision technology is being applied in the transportation sector, three researchers working on related projects were interviewed: Dr. Chengjun Liu, working on Smart Traffic Video Analytics and Edge Computing at the New Jersey Institute of Technology; Dr. Mohammad Jalayer, developing an AI-based Surrogate Safety Measure for intersections at Rowan University, and Asim Zaman, PE, currently researching how computer vision can improve safety for railroads. All researchers expressed that this technology is imminent, effective, and will affect staffing needs and roles at transportation agencies.
A summary of these interviews is presented below.
Smart Traffic Video Analytics (STVA) and Edge Computing (EC) – Dr. Chengjun Liu, Professor, Department of Computer Science, New Jersey Institute of Technology
Dr. Chengjun Liu is a professor of computer science at the New Jersey Institute of Technology, where he leads the Face Recognition and Video Processing Lab. In 2016, NJDOT and the National Science Foundation (NSF) funded a three and-a-half year research project The project led to the development of several promising tools, including a Smart Traffic Video Analysis (STVA) system that automatically counts traffic volume, and detects crashes, traffic, slowdowns, wrong-way drivers, and pedestrians, and is able to classify different types of vehicles.
“There are a number of core technologies involved in these smart traffic analytics.” Dr. Liu said. “In particular, advanced video analytics. Here we also use edge computing because it can be deployed in the field. We also apply some deep learning methods to analyze the video.”
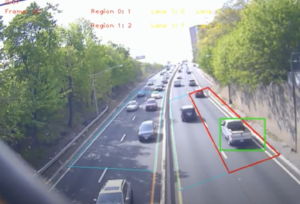
Figure 1. A video feed shows the AI identifying passing vehicles on I-280 in real-time. Courtesy of Innovative AI Technologies.
To test this technology, Dr. Liu’s team developed prototypes to monitor traffic in a real-world setting. The prototype consists of Video Analytics (VA) software, and Edge Computing (EC) components. EC is a computing strategy that seeks to reduce data transmission and response times by distributing computational units, often in the field. In this case, VA and EC systems, consisting of a wired camera with a small computer attached, were placed to overlook segments of both Martin Luther King Jr. Boulevard and I-280 in Newark. Footage shows the device detecting passing cars, counting and classifying vehicles as they enter a designated zone. Existing automated technologies for traffic counting had something in the realm of a 20 to 30 percent error rate, while Dr. Liu reported error rates between 2 and 5 percent.
Additional real-time roadway footage from NJDOT shows several instances of the device flagging aberrant vehicular behavior. On I-280, the system flags a black car stopped on the shoulder with a red box. On another stretch of highway, a car that has turned left on a one-way is identified and demarcated. The same technology, being used for traffic monitoring video in Korea, immediately locates and highlights a white car that careens into a barrier and flips. Similar examples are given for congestion and pedestrians.
“This can be used for accident detection, and traffic vehicle classification, where incidents are detected automatically and in real time. This can be used in various illumination conditions like nighttime, or weather conditions like snowing, raining, and so forth.” Dr. Liu said.
According to Dr. Liu, video monitoring at NJDOT is being outsourced, and it might take days, or even weeks, to review and receive data. Staff monitor operations via video monitors from NJDOT facilities, where, due to human capacity constraints, some incidents and abnormal driving behavior go unnoticed. Like many tools using computer vision, the STVA system can provide live metrics, allowing for more effective monitoring than is humanly possible and accelerating emergency responder dispatch times.
STVA, by automating some manned tasks, would change workplace needs in a transportation agency. Rather than requiring people to closely monitor traffic and then make decisions, use of this new technology would require staff capable of working with the software, troubleshooting its performance, and interpreting the data provided for safety, engineering, and planning decisions.
Dr. Liu was keen to see his technology in use, expressing how the private sector was already deploying it in a variety of contexts. In his view, it was imperative that STVA be implemented to improve traffic monitoring operations. “There is a potential of saving lives,” Dr. Liu said.
Safety Analysis Tool - Dr. Mohammad Jalayer, Associate Professor, Civil and Environmental Engineering, Rowan University
Dr. Mohammad Jalayer, an associate professor of civil and environmental engineering at Rowan University, has been researching the application of computer vision to improving safety at intersections. While Dr. Liu’s STVA technology might focus more heavily on real-time applications, Dr. Jalayer’s research looks to use AI-based video analytics to understand and quantify how traffic functions at certain intersections and, based on that analysis, provide data for safety changes.
Traditionally, Dr. Jalayer said, safety assessments are reactive, “meaning that we need to wait for crashes to happen. Usually, we analyze crashes for three years, or five years, and then figure out what’s going on.” Often, these crash records can be inaccurate, or incomplete. Instead, Dr. Jalayer and his team are looking to develop proactive approaches. “Rather than just waiting for a crash, we wanted to do an advanced analysis to make sure that we prevent the crashes.”
Because 40 percent of traffic incidents occur at intersections, many of them high-profile crashes, the researchers chose to focus on intersection safety. For this, they developed the Safety Analysis Tool.
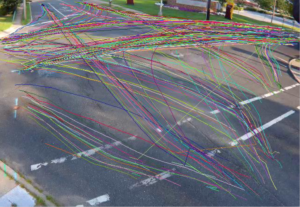
Figure 2. The Surrogate Safety Analysis in action, using user behavior to determine recurring hazards at intersections. Courtesy of Dr. Jalayer.
The Surrogate Safety Measure analyzes conflicts and near-misses. The implementation of a tool like the Surrogate Safety Measure will help staff to make more informed safety decisions for the state’s intersections. The AI-based tool uses a deep learning algorithm to look at many different factors: left-turn lanes, traffic direction, traffic count, vehicle type, and can differentiate and count pedestrians and bicycles as well.
The Safety Analysis Tool’s Surrogate Safety Measure contains two important indicators: Time To Collision (TTC), and Post-Encroachment Time (PET). These are measures of how long it would take two road users to collide, unless further action is taken (TTC), and the amount of time between vehicles crossing the same point (PET), which is also an effective indicator of high-conflict areas.
In practice, these metrics would register, for example, a series of red-light violations, or people repeatedly crossing the street when they should not. Over time, particularly hazardous areas of intersections can be identified, even if an incident has not yet occurred. According to Dr. Jalayer, FHWA and other traffic safety stakeholders have already begun to integrate TTC and PET into their safety analysis toolsets.
Additionally, the AI-based tool can log data that is currently unavailable for roadways. For example, it can generate accurate traffic volume reports, which, Dr. Jalayer said, are often difficult to find. As bicycle and pedestrian data is typically not available, data gathered from this tool would significantly improve the level of knowledge about user behavior for an intersection, allowing for more effective treatments..
In practice, after the Safety Analysis Tool is applied, DOT stakeholders can decide which treatment to implement. For example, Jalayer said, if the analysis finds a lot of conflict with left turns at the intersection, then perhaps the road geometry could be changed. In the case of right-turn conflicts, a treatment could look at eliminating right turns on red. Then, Jalayer said, there are longer-term strategies, such as public education campaigns.
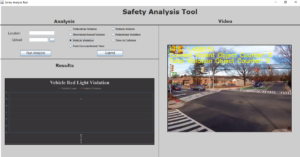
Figure 3. The Safety Analysis tool user interface, which can run various analyses of traffic video, such as vehicle violations, or pedestrian volume. Courtesy of Dr. Jalayer.
For the first phase of the project, the researchers deployed their technology at two intersections in East Rutherford, near the American Dream Mall. For the current second phase, they are collecting data at ten intersections across the state, including locations near Rowan and Rutgers universities.
Currently, this type of traffic safety analysis is handled in a personnel-intensive way, with a human physically present studying an intersection. But with the Surrogate Safety tool, the process will become much more efficient and comprehensive. The data collected will be less subject to human error, as it is not presently possible for staff to perfectly monitor every camera feed at all times of day.
This technology circumvents the need for additional staff, removing the need for in-person field visits or footage monitoring. Instead of staff with the advanced technical expertise to analyze an intersection’s safety in the field, state agencies will require personnel proficient in maintaining the automated equipment.
Many state traffic intersections are already equipped with cameras, but the data is not currently being analyzed using computer vision methods. With much of the infrastructure already present, Dr. Jalayer said that the next step would be to feed this video data into their software for analysis. There are private companies already using similar computer-vision based tools. “I believe this is a very emerging technology, and you're seeing more and more within the U.S.,” Dr. Jalayer said. He expects the tool to be launched by early 2022. The structure itself is already built, but the user interface is still under development. “We are almost there.” Dr. Jalayer said.
AI-Based Video Analytics for Railroad Safety – Asim Zaman, PE, Project Engineer, Artificial Intelligence / Machine Learning and Transportation research, Rutgers University
Asim Zaman, a project engineer at Rutgers, shared information on an ongoing research project examining the use of computer analytics for the purpose of improving safety on and around railways. The rail safety research is led by Dr. Xiang Liu, a professor of civil and environmental engineering at Rutgers Engineering School, and involves training AI to detect trespassers on the tracks, a persistent problem that often results in loss of life and serious service disruptions. “Ninety percent of all the deaths in the railroad industry come from trespassing or happen at grade crossings,” Zaman said.
The genesis of the project came from Dr. Liu hypothesizing that, “There's probably events that happen that we don't see, and there's nothing recorded about, but they might tell the full story.” Thus, the research team began to inquire into how computer vision analysis might inform targeted interventions that improve railway safety.

Figure 4. The color overlay of vehicles trespassing on railways demonstrates that the AI has successfully detected them. Courtesy of Zaman, Ren, and Liu.
Initially, the researchers gathered some sample video, a few days' worth of footage along railroad tracks, and analyzed it using simple artificial intelligence methods to identify “near-miss events,” where people were present on the tracks as a train approached, but managed to avoid being struck. Data on near-misses such as these are not presently recorded, leading to a lack of comprehensive information on trespassing behavior.
After publishing a paper on their research, the team looked into integrating deep learning neural networks into the analysis, which can identify different types of objects. With this technology, they again looked at trespassers, using two weeks of footage this time. This study was effective, but still computationally-intensive. For their next project, with funding from the Federal Railroad Administration (FRA), they looked at the efficacy of applying a new algorithm, YOLO (You Only Look Once), to generate a trespassing database.
The algorithm has been fed live video from four locations over the past year, beginning on January 1, 2021, and concluding on December 31. Zaman noted that, with the AI’s analysis and the copious amounts of data, the research can begin to ask more granular questions such as, “How many trespasses can we expect on a Monday in winter? Or, what time of day is the worst for this particular location? Or, do truck drivers trespass more?”

Figure 5. Similar work shows AI identifying and flagging pedestrian trespassers. The researchers are currently working on using unreported “near-miss” data to improve safety. Courtesy of Zaman, Ren, and Liu.
After the year’s research has concluded, the researchers will study the data and look for applications. Without the AI integration, however, such study would be time-consuming and impractical. The applications fall under the “3E” categories: engineering, education, and enforcement. For example, if the analysis finds that trespassing tends to happen at a particular location at 5pm, then that might be when law enforcement are deployed to that area. If many near-misses are happening around high school graduation, then targeted education and enforcement would be warranted during this time. But without this analysis, no measures would be taken, as near-misses are not logged.
Currently, this type of technology is in the research stage. “We're kind of in the transition between the proof of concept and the deployment here,” Zaman said. The researchers are focused on proving its effectiveness, with the goal of enabling railroads and transit agencies to use these technologies to study particularly problematic areas, and determine if treatments are working or if additional measures are warranted. “It's already contributing, in a very small way, to safety decision making.”
Zaman said that the team at Rutgers was very interested in sharing this technology, and its potential applications, with others. In his estimation, these computer analytics are about five years from a more widespread rollout. He notes that this technology would be greatly beneficial as a part of transportation monitoring, as “AI can make use out of all this data that’s just kind of sitting there or getting rinsed every 30 days.”
Applying computer vision to existing video surveillance will help to address significant safety issues that have persistently affected the rail industry. The AI-driven safety analysis will identify key traits of trespassing that have been previously undetected, assisting decision makers in applying an appropriate response. As with other smart video analytics technologies, the benefit, lies in the enhanced ability to make informed decisions that save lives and keep the system moving.
Current and Future Research
The Transportation Research Board’s TRID Database provides recent examples of how automated video analytics are being explored in a wider context. For example, in North Dakota, an in-progress project, sponsored by the University of Utah, is studying the use of computer vision to automate the work of assessing rural roadway safety. In Texas, researchers at the University of Texas used existing intersection cameras to analyze pedestrian behavior, publishing two papers on their findings.
The TRID database also contains other recent research contributions to this emerging field. The article, “Assessing Bikeability with Street View Imagery and Computer Vision” (2021) presents a hybrid model for assessing safety, applying computer vision to street view imagery, in addition to site visits. The article, "Detection of Motorcycles in Urban Traffic Using Video Analysis: A Review" (2021), considers how automatic video processing algorithms can increase safety for motorcyclists.
Finally, the National Cooperative Highway Research Program (NCHRP) has plans to undertake a research project, Leveraging Artificial Intelligence and Big Data to Enhance Safety Analysis once a contractor has been selected. This study will develop processes for data collection, as well as analysis algorithms, and create guidance for managing data. Ultimately, this work will help to standardize and advance the adoption of AI and machine learning in the transportation industry.
The NCHRP Program has also funded workforce development studies to better prepare transportation agencies for adapting to this rapidly changing landscape for transportation systems operations and management. In 2012, the NCHRP publication, Attracting, Recruiting, and Retaining Skilled Staff for Transportation System Operations and Management, identified the growing need for transportation agencies to create pipelines for system operations and management (SOM) staff, develop the existing workforce with revamped trainings, and increase awareness of the field’s importance for leadership and the public. In 2019, the Transportation Systems Management and Operations (TSMO) Workforce Guidebook further detailed specific job positions required for a robust TSMO program. The report considered the knowledge, skills, and abilities required for these job positions and tailored recommendations to hiring each position. The report compiled information on training and professional development, including specific training providers and courses nationwide.
Conclusion
Following a brief scan of current literature and Interviews with three NJ-based researchers, it is clear that computer vision is a broadly applicable technology for the transportation sector, and that its implementation is imminent. It will transform aspects of both operations monitoring, and safety analysis work, as AI can monitor and analyze traffic video far more efficiently and effectively than human staff. Workplace roles, the researchers said, will shift to supporting the technology’s hardware in the field, as well as managing the software components. Traffic operations monitoring might transition to interpreting and acting on incidents that the Smart Traffic Video Analytics flags. Engineers, tasked with analyzing traffic safety and determining the most effective treatments, will be informed by more expansive data on aspects such as driver behavior and conflict areas than available using more traditional methods.
The adoption of computer vision in the transportation sector will help to make our roads, intersections, and railways safer. It will help transportation professionals to better understand the conditions of facilities they monitor, providing invaluable insight for how to make them safer, and more efficient for all users. Most importantly, these additional metrics will provide ways of seeing how people behave within our transportation network, often in-real time, enabling data-driven interventions that will save lives.
State, regional and local transportation agencies will need to recruit and retain staff with the right knowledge, skills and abilities to capture the safety and operations benefits and navigate the challenges of adopting new technologies in making this transition.
Resources
Center for Transportation Research. (2020). Video Data Analytics for Safer and More Efficient Mobility. Center for Transportation Research. https://ctr.utexas.edu/wp-content/uploads/151.pdf
City of Bellevue, Washington. (2021). Accelerating Vision Zero with Advanced Video Analytics: Video-Based Network-Wide Conflict and Speed Analysis. National Operations Center of Excellence. https://transops.s3.amazonaws.com/uploaded_files/City%20of%20Bellevue%2C%20WA%20-%20Conflict%20and%20Speed%20Analysis%20-%20NOCoE%20Case%20Study.pdf
Espinosa, J., Velastín, S., and Branch, J. (2021). "Detection of Motorcycles in Urban Traffic Using Video Analysis: A Review," in IEEE Transactions on Intelligent Transportation Systems, Vol. 22, No. 10, pp. 6115-6130, Oct. 2021. https://ieeexplore.ieee.org/document/9112620
Ito, Koichi, and Biljecki, Filip. (2021). “Assessing Bikeability with Street View Imagery and Computer Vision.Transportation Research Part C: Emerging Technologies. Volume 132, November 2021, 103371. https://doi.org/10.1016/j.trc.2021.103371
Jalayer, Mohammad, and Patel, Deep. (2020). Automated Analysis of Surrogate Safety Measures and Non-compliance Behavior of Road Users at Intersections. Rowan University. https://www.njdottechtransfer.net/wp-content/uploads/2020/11/Patel-Jalayer-with-video.pdf
Liu, Chengjun (2021). Stopped Vehicle Detection. New Jersey Institute of Technology. https://web.njit.edu/~cliu/NJDOT/DEMOS.html
Liu, X., Baozhang, R., and Zaman, A. (2019). Artificial Intelligence-Aided Automated Detection of Railroad Trespassing. Transportation Research Record: Journal of the Transportation Research Board. https://doi.org/10.1177%2F0361198119846468
Cronin, B., Anderson, L., Fien-Helfman, D., Cronin, C., Cook, A., Lodato, M., & Venner, M. (2012). Attracting, Recruiting, and Retaining Skilled Staff for Transportation System Operations and Management. National Cooperative Research Program (No. Project 20-86). http://nap.edu/14603
Pustokhina, I., Putsokhin, D., Vaiyapuri, T., Gupta, D., Kumar, S., and Shankar, K. (2021). An Automated Deep Learning Based Anomaly Detection in Pedestrian Walkways for Vulnerable Road Users Safety. Safety Science. https://doi.org/10.1016/j.ssci.2021.105356
Szymkowski, T,. Ivey, S., Lopez, A., Noyes, P., Kehoe, N., Redden, C. (2019). Transportation Systems Management and Operations (TSMO) Workforce Guidebook: Final Guidebook. https://transportationops.org/tools/tsmo-workforce-guidebook.
Shi, Hang and Liu, Chengjun. (2020). A New Cast Shadow Detection Method for Traffic Surveillance Video Analysis Using Color and Statistical Modeling. Image and Vision Computing. https://doi.org/10.1016/j.imavis.2019.103863
Upper Great Plains Transportation Institute. (2021). Intelligent Safety Assessment of Rural Roadways Using Automated Image and Video Analysis (Active). University of Utah. https://www.mountain-plains.org/research/details.php?id=566
Zhang, Z., Liu, X., and Zaman, A. (2018). Video Analytics for Railroad Safety Research: An Artificial Intelligence Approach. Transportation Research Record: Journal of the Transportation Research Board. https://doi.org/10.1177%2F0361198118792751
Zhang, T. Guo, M., and Jin, P. (2020). Longitudinal-Scanline-Based Arterial Traffic Video Analytics with Coordinate Transformation Assisted by 3D Infrastructure Data. Transportation Research Record: Journal of the Transportation Research Board. https://doi.org/10.1177%2F0361198120971257