A recently completed research study on NJ TRANSIT grade crossing safety focuses on identifying locations for rail grade crossing elimination. Researchers from Rutgers’ Center for Advanced Infrastructure and Transportation (CAIT), Asim Zaman, P.E., Xiang Liu, Ph.D., and Mohamed Jalayer, Ph.D., from Rowan University, developed a methodology using 20 criteria to narrow a list of 100 grade crossings to ensure appropriate identification for closure. The process helps NJ TRANSIT and New Jersey Department of Transportation (NJDOT) to direct limited funds to areas of greatest need to benefit the public.
Across the country, 34 percent of railroad incidents over the past ten years have occurred at grade crossings. The elimination of grade crossings can improve public safety, decrease financial burdens, and improve rail service to the public.
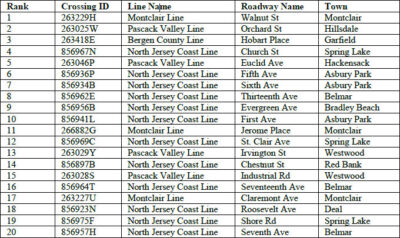
According to the proposed methodology, the 20 crossings recommended for closure located in Monmouth County (60%), Bergen County (25%), and Essex County (25%).
The researchers ranked grade crossings in New Jersey using the following data fields: crash history, average annual daily traffic, roadway speed, roadway lanes, length of the crossing’s street, weekday train traffic, train speed category, number of tracks, access to train platforms, intersection angle, distance to alternate crossings, distance to emergency and municipal buildings, whether emergency and municipal buildings are on the same street, and date of last or future planned signal and surface upgrades. This process resulted in a final list of 20 grade crossings eligible for elimination.
To understand how this study will be used, we conducted an interview with NJTRANSIT personnel Susan O’Donnell, Director, Business Analysis & Research, Ed Joscelyn, Chief Engineer – Signals, and Joseph Haddad, Chief Engineer, Right of Way & Support.
Q. How will the report inform decision-making?
It is important to have solid research and strong evaluation criteria, such as developed by this study, on which to base decisions for grade crossing elimination. In addition to the study, we looked at what other state agencies and transit agencies have done with grade crossing elimination, as well as criteria recommendations from Federal Highway Administration (FHWA) and Federal Railroad Administration (FRA). Following up on this study, NJ TRANSIT and NJDOT are considering next steps that would be needed to close the 20 identified grade crossings. In New Jersey, the Commissioner of Transportation has plenary power over the closing of grade crossings.
Q. What other information will be needed to assess these locations?
Local concerns about grade crossing elimination tend to focus on traffic re-routing, including the possible impacts on neighborhoods, time needed to reach destinations, and emergency vehicle access to all parts of a community. The criteria established by the study addressed these areas of concern. Prior studies have determined that the road networks around the identified locations are adequate to accommodate re-routed traffic. The current research study took into account the findings from those prior studies. As each project moves forward, NJDOT will determine if additional information will be needed.
Q. Is elimination of any of these grade crossings part of NJ TRANSIT’s capital program?
All of the closings are part of the capital program. Funding for the grade crossing elimination comes from the federal government and NJ TRANSIT. NJ TRANSIT funding is in place to close the crossings.
Q. Are there benefits of the research study beyond identification of the 20 grade crossings?
The research study developed the criteria and process for identifying grade crossings for elimination. This framework can be used in the future to assess other grade crossings for possible elimination. NJ TRANSIT is grateful to NJDOT for funding this important research project to improve safety.
For more information on this research study, please see the resources section below.
Resources
Zamin, A., Alfaris, R., Li, W., Liu, Z. Jalayer, M., Hubbs, G., Hosseini, P., Calin, J.P., Patel., S. (2022). NJ Transit Grade Crossing Safety. [Final Report]. New Jersey Department of Transportation, Bureau of Research. Retrieved from https://www.njdottechtransfer.net/wp-content/uploads/2023/02/FHWA-NJ-2022-005.pdf
Liu, Z., Jalayer, M., and Zamin, A. (2022). NJ Transit Grade Crossing Safety. [Technical Brief]. New Jersey Department of Transportation, Bureau of Research. Retrieved from https://www.njdottechtransfer.net/wp-content/uploads/2023/02/FHWA-NJ-2022-005-TB.pdf